Translate this page into:
Building Physician-Scientist Skills in R Programming: A Short Workshop Report
✉Corresponding author email: mhaliyu@yahoo.com
Abstract
Introduction:
Statistical analysis programs require coding experience and a basic understanding of programming, skills which are not taught as part of medical school or residency curricula.
Methods:
We conducted a five-day course for early-career Nigerian physician-scientists interested in learning common statistical tests and acquiring R programming skills. The workshop included didactic presentations, small group learning activities, and interactive discussions. A baseline questionnaire captured participant demographics and solicited participants' level of confidence in understanding/performing common statistical tests. REDCap questionnaires were emailed to obtain feedback on educational format and content. A postworkshop assessment covered participants' overall impression of the program.
Results:
A total of 23 participants attended the program. Most participants were male (n=14, 60.9%) and at an early stage in their career (assistant professor, n=20, 87.0%). Approximately 70% of respondents indicated having received some prior training in statistics. The proportion of participants without experience using R and SAS software (90% and 85%, respectively) was greater than the corresponding proportions for Stata (55%) and SPSS (20%). Prior to the workshop, most respondents expressed being “not at all confident” in performing one-way ANOVA (60%), logistic regression (68%), simple linear regression (60%), and McNemar's test (80%). There was a statistically significant post-workshop improvement in the level of confidence in understanding and performing common statistical tests. The course was rated on a 0-100 scale as “moderately difficult” (mean ± SD: 51.7 ± 19.5). Most participants felt comfortable in putting the knowledge learned into practice (82.2 ± 17.1).
Conclusion and Public Health Implications:
Introductory R can be taught to junior physician-scientists in resource-limited settings and can inform the development and implementation of similar training initiatives in analogous settings.
Keywords
R Programming
Statistical Analysis Training
Physician-Scientists
Low- and Middle-Income Countries
Introduction
To become successful academic researchers, physician-scientists in low- and middle-income countries (LMICs) need to be skilled in the collection, management, analysis, and interpretation of research data. Unfortunately, most statistical analysis programs require coding experience and a basic understanding of programming, skills which are not taught as part of medical school or residency curricula. In addition, popular statistical packages require subscription fees that may not be affordable to LMIC investigators and institutions. R is an open-source, interactive software system that is widely used for data manipulation, computation, analysis, and visualization.1,2
In 2020, the Fogarty International Center (FIC) of the U.S. National Institutes of Health (NIH) funded a training program to build the research capacity of physician-scientists in HIV and non-communicable diseases (NCDs) in Kano, Nigeria. As part of this effort, several workshops were proposed, covering multiple areas of identified training needs.3 One such workshop focused on building physician- scientists' knowledge and proficiency in statistical programming using R. In this article, we describe the key findings from the workshop and post-workshop activities to sustain the impact of training. We also offer recommendations for the development and implementation of similar training models for building capacity in statistical analysis in LMICs globally.
Methods
Background
The parent program for this workshop (Vanderbilt- Nigeria Building Capacity in HIV and NCDs, ‘V-BRCH’) was funded by the FIC/NIH as a platform to create a cohort of skilled Nigerian physician- scientists trained to lead independent clinical trials focused on the intersection of HIV and NCDs.3 The grant was based at the Aminu Kano Teaching Hospital (AKTH) in Kano, Nigeria. As part of the grant, short-term learning opportunities included biannual, on-site, interactive workshops focused on building knowledge and proficiency in essential areas, including clinical trials methodology, evidence synthesis, qualitative and quantitative research methodology, stakeholder engagement, knowledge translation, responsible conduct of research, mentoring and leadership, as well as grant writing.
Workshop Development
The five-day hands-on workshop was held from March 1 – 5, 2021, at the African Center of Excellence in Population Health and Policy at Bayero University in Kano, Nigeria. The course was designed for early-career physician-scientists at AKTH/Bayero University, Nigeria, interested in learning the various fundamental statistical tests commonly used in clinical research settings and acquiring skills to use R in their research endeavors. The curriculum was revised by local investigators to incorporate domestic (Nigeria) considerations. The workshop faculty included two trainers (one Nigeria-born, U.S.-based consultant and an AKTH-based V-BRCH investigator).
The objectives of the workshop were as follows: 1) enable participants to learn how to develop research questions; 2) select the most appropriate statistical test to answer those questions; and 3) operationalize their statistical considerations using R software. At the end of the course, participants were expected to: 1) understand statistical terminology used in clinical research; 2) demonstrate improvement in their level of statistical literacy as applied to clinical research; and 3) exhibit enhanced understanding and proficiency using R software. The course covered basic concepts using interactive, illustrative examples, which were grounded in clinically relevant topics and easily understood. The development of workshop objectives and content was led by the consultant and investigators on the grant, in close collaboration with Vanderbilt-based colleagues.
The workshop targeted early-career physician- scientists (instructor or assistant professor level) at Bayero University and AKTH, Nigeria. The program's website and social media outlets were employed to create demand and generate publicity for the application process. Applicants were requested to apply through an online REDCap link. Candidates were also asked to provide their curriculum vitae and a short statement regarding their interest in attending the workshop and the perceived benefit to them in attending. Applicants were required to obtain permission from their direct supervisor to attend the full five days of the workshop. Applications were reviewed by a team of five V-BRCH investigators and a program manager. Priority was given to applicants who met the above criteria and were enrolled in or were alumni of other NIH/Fogarty-funded training programs at AKTH, as this demonstrated further evidence of their commitment to a research/academic career.
Workshop Outline and Implementation
The workshop was divided into five modules and included didactic presentations, small group learning activities, and interactive discussions. The first three modules (days 1-3) covered study design, statistical concepts, and t-tests. The topics for each module were selected based on relevance to the module and appropriateness to the workshop goals. For instance, module 1 (study design) covered levels of evidence, case-control and cross-sectional studies, cohort study designs, experimental study designs, validity in epidemiologic studies (bias, confounding, and effect modification), dimensions of data quality, and screening tests. The last two modules (days 4 and 5) included ANOVA, correlation, simple linear regression, Chi- square, Fisher's exact test, McNemar's test, and logistic regression. The afternoon small groups' hands-on R sessions were focused on learning the R interface, how to upload datasets, save programs, write programming codes, and run R scripts efficiently. Participants were also trained in performing the statistical tests covered in didactic sessions in R and interpreting the results. These sessions were primarily comprised of activities that emphasized hands-on skills acquisition.
Evaluation
Participants were notified of their selection for the workshop by email. A link to a structured preworkshop questionnaire was included in the email. The baseline questionnaire captured information on participant demographics and solicited participants' level of confidence (Likert scale, 1 = not confident, 3 = very confident) in understanding and performing selected statistical tests, specifically t-test, one-way ANOVA, correlation, simple linear regression, Chi- square test, Fisher's exact test, McNemar's test, and logistic regression. Participants were also asked to rank their level of comfort (no experience, somewhat comfortable, or very comfortable) in using R and three other common statistical software packages, namely SPSS, SAS, and STATA.
REDCap questionnaires were emailed at the end of each workshop day to obtain in-depth, real-time feedback from course participants. Participants were asked to rate each session based on educational content, instructor's knowledge of the subject matter, quality of the presentation, time for discussion, and perceived usefulness of the session (5-item Likert scale, 1 = poor and 5 = excellent). A post-workshop assessment covered participants' overall impression of the training program and solicited open-ended responses. All evaluations were confidential. A program manager summarized the evaluation results at the end of the workshop. Ethical approval for the program was obtained from the Vanderbilt University Institutional Review Board and the Ethics Review Committee at AKTH, Nigeria.
Results
A total of 23 participants attended the program (Table 1). All participants except one were faculty members from AKTH/Bayero University. Most participants were male (n = 14, 60.9%), at an early stage in their career (assistant professor level, n = 20, 87.0%), and drawn from adult medicine (n = 7), laboratory sciences (n = 5), and pediatrics departments (n = 5).
Characteristic | Number | % |
---|---|---|
Sex | ||
Female | 9 | 39.1 |
Male | 14 | 60.9 |
Specialty | ||
Clinical research | 1 | 4.4 |
Dentistry | 1 | 4.4 |
Laboratory sciences | 5 | 21.7 |
Medicine | 7 | 30.4 |
Pediatrics | 5 | 21.7 |
Public health | 1 | 4.4 |
Surgical specialties | 3 | 13.0 |
Academic Rank | ||
Assistant Professor | 20 | 87.0 |
Associate Professor | 2 | 8.7 |
Other | 1 | 4.4 |
Laboratory sciences: chemical pathology, clinical pathology, hematology; Medicine: cardiology, endocrinology family medicine, infectious diseases, neurology, nephrology; Pediatrics: pediatric nephrology, pediatric neurology, pediatric infectious diseases; Surgical specialties: cardiothoracic surgery, gastrointestinal surgery, radiology
Twenty participants responded to both the pre-and post-workshop surveys (response rate = 87%). Approximately 70% of respondents indicated having received some prior training in statistics (course, workshop, etc.) (Table 2). The proportion of participants without experience using R and SAS software (90% and 85%, respectively) was much greater than the corresponding proportions for STATA (55%) and SPSS (20%). More than half of the participants (60%) reported being somewhat comfortable using SPSS (Table 2).
Topic | N=20 | |
---|---|---|
Prior training in statistics (including courses, workshops, etc.) | ||
Yes | 70% | |
No | 30% | |
Level of comfort using R | ||
No experience | 90% | |
Somewhat comfortable | 5% | |
Very comfortable | 0% | |
Missing | 5% | |
Level of comfort using SAS | ||
No experience | 85% | |
Somewhat comfortable | 5% | |
Very comfortable | 0% | |
Missing | 10% | |
Level of comfort using Stata | ||
No experience | 55% | |
Somewhat comfortable | 30% | |
Very comfortable | 10% | |
Missing | 5% | |
Level of comfort using SPSS | ||
No experience | 20% | |
Somewhat comfortable | 60% | |
Very comfortable | 20% |
Prior to the workshop, we assessed respondents' level of confidence in performing various statistical tests (Figure 1). More than half of the respondents expressed being “not at all confident” in performing one-way ANOVA (60%), logistic regression (68%), simple linear regression (60%), and McNemar's test (80%). Participants were also surveyed before and after the workshop regarding their level of confidence (rated 1-3) in understanding and performing common statistical tests using R (Table 3). There was a statistically significant improvement in the level of confidence in understanding and performing all ten statistical tests. The largest improvement (100% increase in the mean score) was noted for McNemar's test, followed by paired sample t-test (61%), one-way ANOVA (61%), and logistic regression (60%) (Table 3).
One Sample t-test | Two Sample t-test | Paired Sample t-test | One-way ANOVA | Correlation | Simple Linear Regression | Chi-square Test | Fisher's Exact Test | McNemar's Test | Logistic Regression | |
---|---|---|---|---|---|---|---|---|---|---|
Pre-survey | ||||||||||
Mean | 2.2 | 2.0 | 1.8 | 1.8 | 1.9 | 1.7 | 2.5 | 2.2 | 1.3 | 1.5 |
Standard | 0.5 | 0.6 | 0.7 | 0.8 | 0.7 | 0.7 | 0.6 | 0.8 | 0.6 | 0.6 |
Deviation | ||||||||||
Post-survey | ||||||||||
Mean | 2.9 | 2.8 | 2.9 | 2.9 | 2.8 | 2.7 | 2.9 | 2.9 | 2.7 | 2.4 |
Standard | 0.5 | 0.5 | 0.5 | 0.5 | 0.5 | 0.5 | 0.3 | 0.4 | 0.6 | 0.5 |
Deviation | ||||||||||
Change in mean score (%)* | 32 | 40 | 61 | 61 | 47 | 59 | 16 | 32 | 100 | 60 |
Paired sample t-test, P-value | <0.0001 | 0.0002 | <0.0001 | <0.0001 | 0.0003 | <0.0001 | 0.009 | 0.0009 | <0.0001 | <0.0001 |
*Percent change in mean score was calculated as follows: [(post-survey mean – pre-survey mean) ÷ (pre-survey mean)] × 100
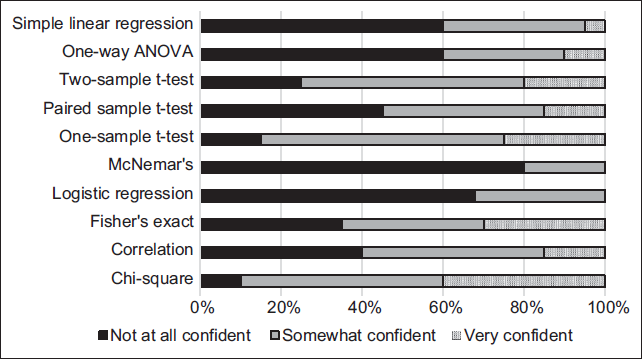
- Level of Confidence in Performing Specific Statistical Analyses at Baseline
The post-workshop survey requested trainees to rate the effectiveness of the instructor and the difficulty, organization, and overall quality of the course (Table 4). Nearly all respondents rated the course and effectiveness of the instructor as “excellent” (90% and 95%, respectively). Whereas the overall course was rated on a 0-100 scale as “moderately difficult” (mean ± SD: 51.7 ± 19.5), the trainees felt the course was highly organized (89.5 ± 10.3), and the R software program was relatively easy to learn (80.7 ± 18.9). The overwhelming majority of respondents felt comfortable in putting the knowledge learned into practice (82.2 ± 17.1). All respondents indicated that they would be “very likely” to recommend the course to fellow clinical researchers (100%).
N=20 | |
---|---|
Effectiveness of instructor | |
Excellent | 95% |
Average | 5% |
Difficulty of the course | |
Mean | 51.7 |
Standard Deviation | 19.5 |
Organization of the course | |
Mean | 89.5 |
Standard Deviation | 10.3 |
Ease of learning R software | |
Mean | 80.7 |
Standard Deviation | 18.9 |
Level of comfort in putting R knowledge into practice | |
Mean | 82.2 |
Standard Deviation | 17.1 |
Overall rating of the course | |
Excellent | 90% |
Good | 5% |
Average | 5% |
Likelihood of recommending the course to other clinical researchers | |
Very Likely | 100% |
Discussion
We herein describe results from a workshop in Nigeria to train junior physician-scientists to learn how to develop research questions, select the most appropriate statistical test to answer those questions, and operationalize these statistical methods using R software. Prior studies suggest that trainees can learn R without having a robust background in statistics.4 Although 70% of our respondents indicated having received some level of prior training in statistics, the overwhelming majority (90%) had no experience using R software, justifying the need for the training. Our finding of a statistically significant improvement in the level of confidence in understanding and performing statistical tests is consistent with the notion that statistical software (such as R) is valuable in teaching statistics in medical education and can be appreciated by persons without a priori knowledge of programming.5
It is not surprising that more than half of our respondents expressed being “not at all confident” in performing regression analyses (one-way ANOVA, logistic regression, simple linear regression). The Nigerian medical school curriculum limits the scope of biostatistics instruction to hand calculation of formulas underlining basic univariate analyses, such as Chi-square and Student's t-test. Regression methods would be difficult to demonstrate and comprehend using manual approaches. Despite their relatively low confidence level in conducting statistical analyses at baseline, at the conclusion of the program, 90% of participants rated the workshop as “excellent,” and all participants indicated that they would be “very likely” to recommend the course to other clinical researchers. Our results are consistent with Baumer et al., who found that a lack of having prior coding experience did not impede the performance or reported satisfaction of students attending a semester-long undergraduate course in R.6
As an open-source tool, R software has affordability advantages over subscription-based platforms like SPSS and SAS, especially in LMICs such as Nigeria. Other advantages of R include its flexibility in permitting exploratory data analyses, interactive data analysis, documentation and reproducibility, quick visualization of data, and the considerable power of numerous packages that expand its data functionality.7,8 The steep learning curve associated with the use of R has been lessened by the advent of development environments such as RStudio, which have decreased the difficulty faced by learners without programming experience.5
The learning and retention of programming skills require continuous practice. A novel feature of our program was the creation of an interactive WhatsApp user group comprising workshop participants, the course instructor, and an experienced U.S.-based R programmer. Following the workshop, this group has voluntarily continued to meet via Zoom every other weekend to explore R-related data analysis scenarios, share data scripts, provide peer support, and facilitate co-learning. Several manuscripts based on local (Nigeria) data are currently in preparation, based on the creation of this novel post-course learning tool. If sustained, this resource will ensure that skills and knowledge learned during the workshop are maintained well beyond the duration of the workshop.
Our study has limitations. The relatively small sample size and participants were drawn from mostly one institution limit the generalizability of our findings. The absence of a comparison (control) group also limits our ability to infer causality in the association between the intervention (training) and changes in the level of confidence in comprehension or performance of specific statistical tests or analyses. Nevertheless, our findings indicate that introductory R can be taught to junior scientists in an LMIC setting and can inform the development and implementation of similar training initiatives in analogous settings. Future research could explore the inclusion of a larger sample size of trainees, multiple sites, and a comparison group of participants.
Compliance with Ethical Standards
Conflicts of Interest:
No conflict of interest to declare.
Financial Disclosure:
Nothing to declare.
Ethics Approval:
Ethical approval for the program was obtained from the Vanderbilt University Institutional Review Board and the Ethics Review Committee at AKTH, Nigeria.
Disclaimer:
The content is solely the responsibility of the authors and does not necessarily represent the official position of the National Institutes of Health.
Acknowledgments:
None.
Funding:
This work was supported by the Fogarty International Center and the National Institute of Alcohol Abuse and Alcoholism of the National Institutes of Health under award number D43 TW0II544.
References
- Data analysis using R programming. Adv Exp Med Biol. 2018;1082:47-122.
- [CrossRef] [PubMed] [Google Scholar]
- An Overview of R in health decision sciences. Med Decis Making. 2017;37(7):735-746.
- [CrossRef] [PubMed] [Google Scholar]
- The V-BRCH Project: building clinical trial research capacity for HIV and noncommunicable diseases in Nigeria. Health Res Policy Syst. 2021;19(1):32.
- [CrossRef] [PubMed] [Google Scholar]
- Teaching R in the undergraduate ecology classroom: approaches, lessons learned, and recommendations. Ecosphere. 2020;11(4):e03060.
- [CrossRef] [Google Scholar]
- Teaching introductory statistical classes in medical schools using RStudio and R statistical language: evaluating technology acceptance and change in attitude toward statistics. J Stat Educ. 2020;28(2):212-219.
- [CrossRef] [Google Scholar]
- R markdown: integrating a reproducible analysis tool into introductory statistics. TechnoI Innov Stat Educ. 2014;8(1):1-29.
- [CrossRef] [Google Scholar]
- An introduction to R. Notes on R: a programming environment for data analysis and graphics. Version 2.6.0 (2007-10-03) (accessed )
- Data analysis in medical research: from foe to friend. Croat Med J. 2019;60(1):1.
- [CrossRef] [PubMed] [Google Scholar]