Translate this page into:
AI-Enhanced Epidemiology Education: Bridging Technology and Conceptual Understanding
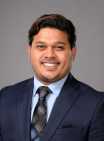
*Corresponding author: Akshaya S. Bhagavathula, Department of Public Health, North Dakota State University, Dept 2662, Fargo, ND, USA. akshaya.bhagavathula@ndsu.edu
-
Received: ,
Accepted: ,
How to cite this article: Bhagavathula AS, AI-enhanced epidemiology education: Bridging technology and conceptual understanding. Int J Transl Med Res Public Health. 2024;8:e011. doi:10.25259/IJTMRPH_55_2024.
Abstract
This article explores the integration of artificial intelligence (AI) and innovative teaching methods in epidemiology education, emphasizing a balanced approach to enhance learning outcomes. While traditional teaching focuses on core concepts and rubric-based evaluation offers consistency, overreliance on these methods can lead to superficial understanding. Creative and experiential learning activities, such as poems, cartoons, and ungrading, foster deeper engagement and mastery. Technological tools such as simulated outbreak investigations, virtual epidemiology games, and augmented reality programs provide hands-on experience and inspire motivated learning. Emphasizing critical thinking and practical skills, a balanced approach leveraging rubrics, AI, and innovative methods is essential for comprehensive and effective epidemiology education.
Keywords
Artificial Intelligence
Epidemiology
Public Health
Education
Teaching Methods
Learning
Technological Innovations
Epidemiology occupies a vital space in public health curricula, equipping students with competencies for assessing and addressing population health issues. Competency development relies on comprehension and application of key causal, statistical, and methodological concepts underpinning epidemiologic practice. For instance, students in Master of Public Health programs with a concentration in epidemiology learn to develop appropriate study designs, interpret epidemiologic data, and communicate findings accurately.[1] Effective teaching focuses on imparting an understanding of core concepts such as disease frequency and association measures, designing valid and ethical observational and experimental studies, and identifying and addressing biases. Assessment through problem sets, reports, and exams should emphasize retaining core epidemiology principles and integrating insights across disciplines.[2]
The COVID-19 pandemic necessitated a rapid transition to online education, highlighting the importance of rubric-based evaluation as a vital assessment tool. Rubrics outline grading criteria and standards, facilitating efficient grading and enhanced reliability across large remote classes.
They serve as “scoring sheets” for learning tasks, articulating evaluation criteria and performance levels. Whether in online or face-to-face courses, rubrics simplify providing feedback and consistent grades to students.
However, the integration of artificial intelligence (AI) into education presents challenges in balancing technological advancements with genuine learning process.[3] In epidemiology, which requires a deep conceptual understanding to design studies, analyze data, interpret findings, and infer causality, overreliance on rubrics and AI tools may prioritize point earning over comprehensive understanding. Similarly, enhanced writing tools, when heavily reliant on inputs like rubric descriptors and exemplar essays, may generate well-articulated but shallow responses, lacking the depth indicative of mastery, and can potentially lead to superficial learning outcomes.
This poses grading challenges but presents opportunities for novel teaching methods that motivate deep investment in core topics. One promising direction is the increased use of innovation-based activities and evaluation tailored to elucidate students’ internalization of key lessons.[4] For instance, students creating poems, cartoons, or mini-plays to convey their understanding of epidemiologic concepts provide imaginative spaces often lacking in conventional assignments. Even traditional writing forms, like epidemic detective stories, can move learning beyond technical replication toward perspective-changing engagement.
Another innovative technique in healthcare education is the “ungrading” approach, where fixed grading systems are replaced with student-directed goal-setting, self-evaluation of progress, and qualitative instructor feedback.[5] This approach fosters a more personalized learning experience, allowing students to take ownership of their learning process and engage more deeply with the materials.
Using varied forms of concepts and mind mapping has been recognized as a valuable technique to promote relational understanding in the field of epidemiology. These visual tools are particularly conducive to epidemiology’s interlinked concepts, as they can elicit connections that students may struggle to explicate in writing.[6] Visual mapping techniques, such as mind and concept mapping, have been found to be effective in promoting meaningful learning and facilitating rational thinking in medical education activities. They allow students to recognize the intra- and interrelationships between concepts, reflecting the kind of real-world thinking predominant in the clinical setting. Furthermore, using interactive online visualization tools enables efficient collaborative construction, which may allow students to work together to create and share visual representations of epidemiologic concepts. These tools can be particularly beneficial in remote or online contexts, as they facilitate collaborative learning and exploration of complex epidemiological interrelationships.
Technological mediums, such as simulated outbreak investigations, virtual epidemiology games, and augmented reality programs, support experiential learning in epidemiology.[7] These innovative applications scaffold case investigations, surveillance, study methodology, and biostatistics competencies, providing hands-on experience in a virtual environment. Augmented reality programs allow manipulating simulated datasets to instantly view impacts “in situ.” Such learning-by-doing simulations inspire intrinsically motivated understanding key for recall and transferability.
Specific examples of AI’s application in public health programs include AI-driven predictive analytics used to anticipate disease outbreaks, enabling more efficient allocation of healthcare resources and vaccination campaigns.[8] Machine learning models have also been employed to analyze social determinants of health, providing policymakers with data-driven insights to design targeted interventions for vulnerable populations. During the COVID-19 pandemic, AI tools were instrumental in real-time syndromic surveillance, helping public health authorities monitor disease spread and implement timely measures.[9]
While the use of rubric and AI in education has their benefits, a balanced approach that leverages the benefits of rubric, AI, and innovative teaching methods is essential to foster a comprehensive and engaging learning environment in epidemiology. This approach should prioritize critical thinking, problem-solving, and practical skills to deepen the mastery of epidemiology required in practice.
Potential research directions
Future research is needed to explore the long-term effects of AI and innovative pedagogies on learning outcomes in epidemiology education. Future studies could employ a mixed- methods approach, combining quantitative assessments of knowledge retention with qualitative evaluations of student engagement and conceptual understanding. Additionally, systematic reviews and meta-analyses of existing studies on AI-enhanced education could provide valuable insights into best practices and potential areas for improvement.
Limitations
While this commentary highlights the potential of AI and innovates teaching methods, it is important to acknowledge the limitations. The rapid adoption of technology in education can sometimes outpace the development of appropriate pedagogical frameworks. Additionally, the effectiveness of these methods may vary depending on the students’ prior knowledge, technological literacy, and access to resources. The COVID-19 pandemic indeed changed the way we see and do things, and while it has accelerated the adoption of technology in education, it also underscored the need for thoughtful implementation to avoid superficial learning outcomes.
CONCLUSION AND IMPLICATIONS FOR TRANSLATION
Emerging education technologies offer efficiency benefits, yet their true potential lies in fostering adaptive mastery of complex epidemiologic concepts. Achieving this requires a balanced approach that integrates technological tools with creativity-inspiring pedagogies, promoting rigorous analysis, engaged skill-building, and curiosity-driven learning. Moving forward, it’s essential to prioritize comprehensive over mere technical proficiency, ensuring that these innovations contribute to the development of ethical, transferable skills. Further research will be critical in guiding best practices at this pivotal juncture, reinforcing the vital role of epidemiology in enhancing community well-being.
Key Messages
Artificial intelligence (AI) and innovative teaching methods offer significant potential to enhance epidemiology education by bridging the gap between technology and conceptual understanding.
A balanced approach that integrates rubric-based evaluation, AI, and creative pedagogies is essential to foster deep learning and mastery of epidemiologic concepts.
Further research is needed to explore the long-term effects of these educational innovations on student outcomes.
Acknowledgments
None
COMPLIANCE WITH ETHICAL STANDARDS
Conflicts of Interest
The authors declare no competing interests.
Financial Disclosure
Nothing to declare.
Funding/Support
There was no funding for this study.
Ethics Approval
Not Applicable.
Declaration of Patient Consent
Not Applicable.
Use of Artificial Intelligence (AI)-Assisted Technology for Manuscript Preparation
The authors confirm that there was no use of artificial intelligence (AI)-assisted technology for assisting in the writing or editing of the manuscript, and no images were manipulated using AI.
Disclaimer
None.
Financial support and sponsorship
Nil.
REFERENCES
- Using transitional year milestones to assess graduating medical students’ skills during a capstone course. J Grad Med Educ.. 2015;7(4):658-62. Dec
- [CrossRef] [Google Scholar]
- An effectiveness evaluation of a community-based course for medical students: A randomized controlled trial in the teaching of epidemiology. BMC Med Educ.. 2023;23(1):807. Oct 27
- [CrossRef] [Google Scholar]
- Teaching epidemiology at the undergraduate level: Considerations and approaches. Am J Epidemiol.. 2018;187(6):1143-8. Jun 1
- [CrossRef] [PubMed] [Google Scholar]
- “It made me feel like it was okay to be wrong”: Student experiences with ungrading. Active Learn High Educ.. 2022;25(1):67-80.
- [CrossRef] [Google Scholar]
- Assessing university students’ critical online reasoning ability: A conceptual and assessment framework with preliminary evidence. Front Educ.. 2020;15(5) Dec 15
- [CrossRef] [PubMed] [Google Scholar]
- Making outbreak investigations real to learners. Med Sci Educ.. 2023;33(2):443-9. Feb 21
- [CrossRef] [Google Scholar]
- Healthcare sustainability: Hospitalization rate forecasting with transfer learning and location-aware news analysis. Sustainability. 2023;15(22)
- [CrossRef] [PubMed] [Google Scholar]
- COVID-19 symptoms app analysis to foresee healthcare impacts: Evidence from Northern Ireland. Appl Soft Comput. 2022:116. Feb
- [CrossRef] [PubMed] [Google Scholar]